VALD Injury Prediction Statement
Available in:
EN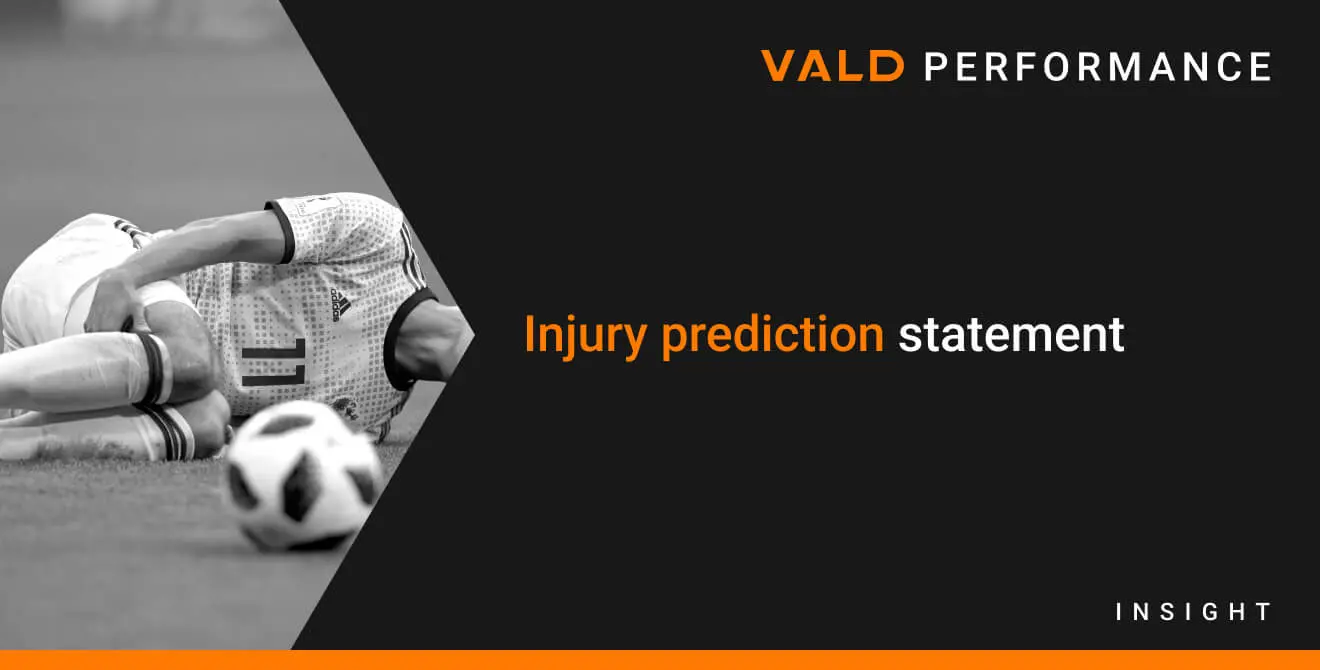
Background
Musculoskeletal injuries typically result from complex, non-linear interactions between multiple factors (Bittencourt et al. 2016). Some factors are non-modifiable, such as age, sex, ethnicity, and training history while others, including strength, muscle-tendon morphology, and diet can be modified. Identifying risk factors for injury represents the first step towards designing evidence-based injury prevention programs.
Injury prediction is the holy grail of sports medicine, but we are a long way from doing this with any degree of accuracy. Philosophically, the aim of predictive modelling is not to ‘predict injury’. Rather, practitioners want to estimate risk at an individual athlete or patient level and then intervene (e.g. via targeted training) to reduce that level of risk.
In prospective studies, researchers measure certain factors in a population, track injury occurrence over a known period, and examine which factors associate – or, in other words, correlate – with injury. If a strong association is observed between one or more measured factors and subsequent injury, it may be tempting to conclude that these factors can be used to predict who is at risk of future injury. However, these models often fail to predict injuries in new populations, or populations that differ in any way from those in the original study because the factors often have no relationship to the mechanism of injury.
While clinical prediction models have traditionally been built with regression-based approaches, machine learning methods are becoming increasingly popular. The latter are often termed ‘black box’ prediction methods because they are impossible to reproduce (Bullock et al. 2022). Machine learning (e.g. gradient boosting, tree-based) and deep learning (e.g. artificial neural networks) has the potential to help unravel the complex non-linear interactions between risk factors – but need to be used appropriately.
Association versus prediction
Practitioners and other companies selling commercial technology frequently confuse association with prediction. A poor understanding of the differences between association and prediction may result in practitioners concluding that a factor associated with injury risk can be used to predict (and ultimately prevent) injury. As a result, companies that use black box prediction methods and claim to predict or prevent injuries have come under increasing scrutiny.
Prospective studies examining ‘associations’ are valuable for identifying risk factors for injury at a group level, and can help us to understand why injury occurs, either directly or indirectly. However, association does not equal causation, which is required to accurately predict injury.
Prediction is the ability to predict a future outcome at an individual level. Because black box methods are rarely built using expert knowledge and clinical reasoning, factors with no causal relevance may be included in model development.
We might observe an increase in the risk of sustaining an injury with an increase in the number of goals scored from penalties during a soccer match. However, reducing the number of goals scored from penalties by recruiting a world-class goalkeeper likely will not reduce the risk of injury. Injury risk, as well as the number of goals scored from penalties, will instead be directly associated with the number of dangerous tackles for which the referee calls a penalty.
“Currently, there is no screening test available to predict sports injuries with adequate test properties and no intervention study providing evidence in support of screening for injury risk.” (Bahr et al. 2016)
Limitations of current machine learning approaches for modelling sports injury
Without full transparency of reporting and the complete presentation of all model equations or code, prediction methods become black boxes.
Black box models cannot aid in interpretation or guide intervention as it is impossible to determine their validity, performance, and clinical utility. The following list summarizes common problems with creating black box models:
- Most sport organizations do not sustain enough injuries within a given season, or multiple seasons, to develop or externally validate a model accurately.
- Models are prone to overfitting, which means they are only useful to predict injuries in the exact same population the models were trained on. Models with predictive accuracy of r= 0.99 should be interpreted with caution as they are likely overfitted. Internal validation should be performed to obtain an unbiased assessment of model performance (e.g. bootstrapping or cross-validation). Bullock et al. recommend avoiding splitting data into development (‘training’) and validation (‘test’) data because it reduces sample size and increases risk of overfitting.
What this means at VALD
Interpret any black box predictive models with caution.
At VALD, we aim to be as transparent as possible about our data processing methods and never suggest that data from our products can be used to predict injury. Instead, we recommend practitioners use our data in combination with other information about the individual athlete, patient or soldier to estimate individual injury risk and develop an appropriate training intervention (e.g. exercise prescription) to reduce that risk.
We will develop smarter predictive analytics in our software, but they will only be used to guide decision-making and never used to replace clinical reasoning.
References
- Bittencourt et al. 2016 British Journal of Sports Medicine
- Bullock et al. 2022 Sports Medicine